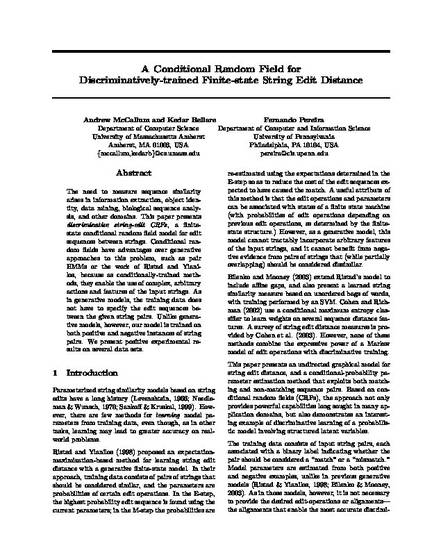
The need to measure sequence similarity arises in information extraction, object identity, data mining, biological sequence analysis, and other domains. This paper presents discriminative string-edit CRFs, a finitestate conditional random field model for edit sequences between strings. Conditional random fields have advantages over generative approaches to this problem, such as pair HMMs or the work of Ristad and Yianilos, because as conditionally-trained methods, they enable the use of complex, arbitrary actions and features of the input strings. As in generative models, the training data does not have to specify the edit sequences between the given string pairs. Unlike generative models, however, our model is trained on both positive and negative instances of string pairs. We present positive experimental results on several data sets.
Available at: http://works.bepress.com/andrew_mccallum/13/